The project is directed toward modeling and simulation of rejections, blocking and utilization problems in ICU suite in the hospital according to the project’s functional specifications. It covers the management of unit capacities in the suite, considering the machine failures, different type of patient arrivals, deaths, rejections and blocking in the units. The project’s objective is to determine the number of beds in each unit in ICU suite so that the rejection probabilities, blocking probabilities and unit utilizations are maintained at a desired level.
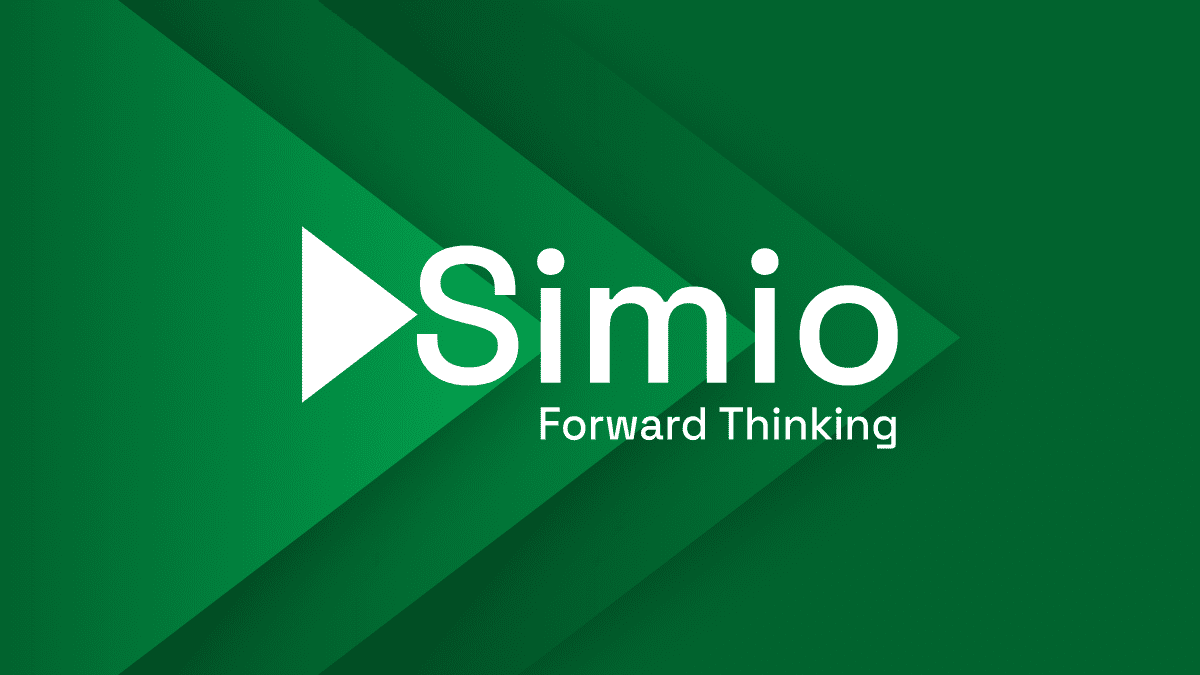
Pitt IE Hospital ICU Suite Simulation Report
INDUSTRY
Healthcare
AUTHOR(S)
M. Ulukus & G. Degirmenci
ORGANIZATION
University of Pittsburgh
Introduction
Summary and Conclusions
When we carefully investigate the impact of capacity changes on the utilization of units, blocking probabilities and blocking times, and the rejection probabilities, we observe that increasing the capacities of both SD unit and telemetry unit, decreases the ICU blocking and SD blocking at a significant level. Moreover, rejection probability is also decreased to 12%. We observe that increasing the capacity of ICU unit, would not decrease the rejection probability significantly, since it causes blocking in the ICU unit. Moreover, increasing the capacities of all units might be costly in terms of both of cost of beds and machines and also cost of the unit usage, since utilities decrease if we increase the capacities of all units. Consequently, we decide that Scenario G, which has 8 beds in ICU, 6 beds in SD unit and 5 beds in Telemetry Unit, is the best scenario that decrease the blocking probability to 4%, 14%, 3% and 33% at ICU, SD, OR and ASR units. One should note that the blocking at ICU is the most important measure, since the cost of beds at ICU is larger than the others and the blocking is a measure of the improper usage of resources at ICU. Moreover, with scenario G, we also maintain the utilization of units at a desired level. The utilizations are 75%, 51% and 55% at ICU, SD and Telemetry units respectively. Increasing the utilization of ICU unit by changing the capacities increases the blocking in ICU. Increasing the utilization in other units also increases the blocking in all departments. Hence, scenario G is the best scenario among the others. In addition to the mean comparisons, half widths support the reliability of the analysis.
More Student Projects
Expansion Project for an Airport Security Area
January 18, 2024
Resiliency of the Pittsburgh International Airport
January 18, 2024
Increasing Customer Satisfaction At Antalya Airport
January 18, 2024