2022 Simio Sync: Practical Applications
WHEN
February 28, 2022
Presentation Highlights & Replays
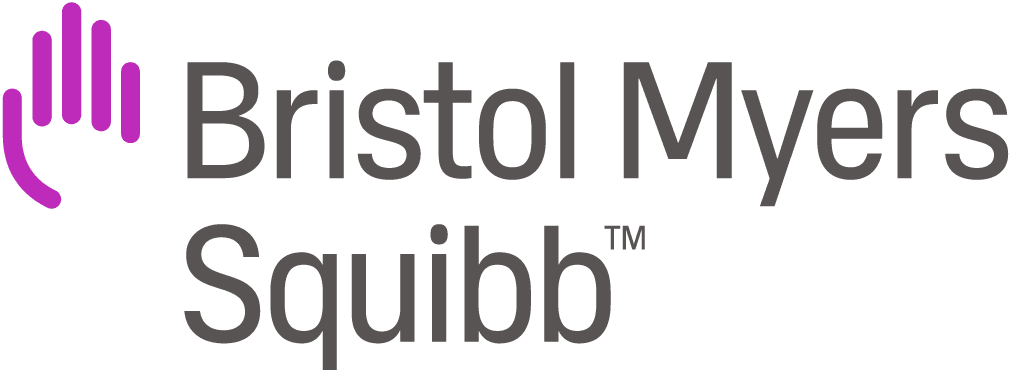
Using Simio to Predict Bottlenecks in Cell Therapy Manufacturing
Presented by Kirill Dobrodomov, Operational Excellence Engineer
In the world of autologous cell therapy manufacturing, where a patient’s own cells are used to treat disease, there are very few conditions that remain constant. The manufacturing process is uniquely complex, time-sensitive, and evolving. In this presentation, we show how Simio is used at a CAR-T manufacturing site to quickly make sense of variable and uncertain inputs, while building toward an accurate and predictable model layer by layer. We use a combination of dashboard reports and Gantt views within Simio to automatically visualize and verify the behavior of the model, allowing us to experiment with inputs efficiently.
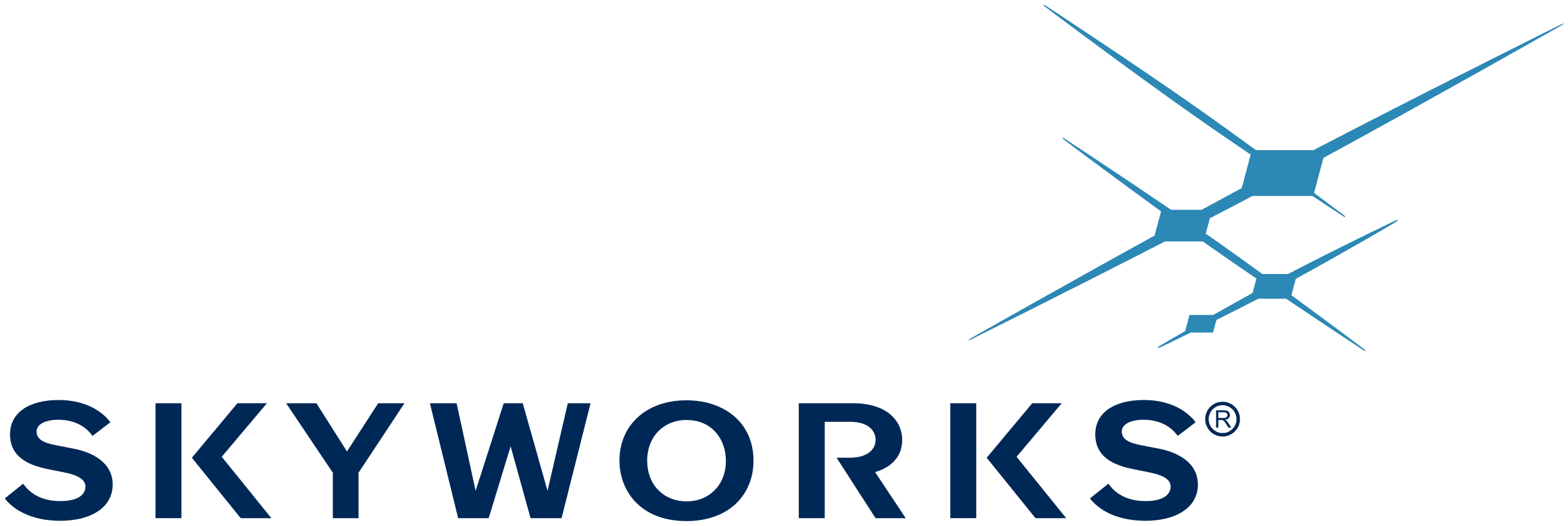
Job Shop Model | Characterization Lab
Presented by Carlos Lares, Sr. BU Ops Manager
Estimating the capacity of a Characterization Lab can be challenging, especially when people have multiple skillsets and test setups with numerous applications, not to mention a very dynamic demand mix with different processing recipes. We applied the typical static capacity calculations using an excel sheet and little’s law. The problem arose when the business asked if we could meet the demand of a potential scenario. The excel model didn’t meet the expectations as we couldn’t determine how the resources would interact without relying on historical data. At this point, we decided to evaluate a modeling software, Simio. With the help of the Simio software and programming assistance services, we constructed a model that simulates how the Characterization Lab could respond if presented with a different scenario. After a few months of collaboration, we modeled the interaction between technicians, setups, and demand, leaving us with a model to answer management’s questions.
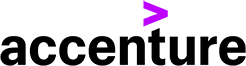
Warehouse Digital Twin
Presented by Ana Ruiz, Data Scientist Consultant Maria Servitja Robert, Data Scientist Manager
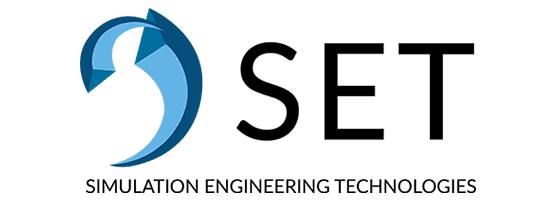
Data-driven modeling for moveable asset logistics
Presented by Handré Dreyer, Senior Solutions Manager
Developing simulation models for movable asset operations (haul trucks, loaders, shovels, etc.) in heavy industries such as mining, is complex and time-consuming. There are many dependencies on multiple systems and data sources, as well as interactions with overall operations.
Fleet management systems monitor and record operational data on movable assets. However, the data is collected in real-time but only viewed in hindsight, resulting in reactive operations. The analysis of the collected data can provide valuable insights on utilisation, availability, idle time, etc. but lacks real-time responsiveness. Therefore, it falls short when it comes to scheduling, planning, target adherence and overall system performance.
By integrating data-driven simulation technologies with fleet management systems, the simulation model will become a predictive analytics tool, incorporating historical and real-time data. The integrated solution can support performance optimisation, provide accurate planning and scheduling, track target adherence, support proactive asset management, and improve cost management.
With the proprietary data-driven modelling approach, the simulation model uses fleet management data to automatically build the simulation model, inclusive of the haulage network. This approach enables a standardised methodology to be applied and reduces the time and costs associated with the construction of such models. Complex model logic is replaced with our proprietary approach, which is fully driven by operational data using the Simio® Simulation Software.
This session showcases how such technology can bring the industry a step closer to benefitting from the Digital Twin.
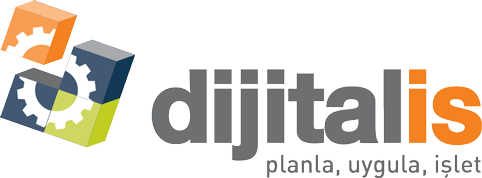
Investment Planning with Long Term Capacity Simulation
Presented by Tolga Yanasik, President
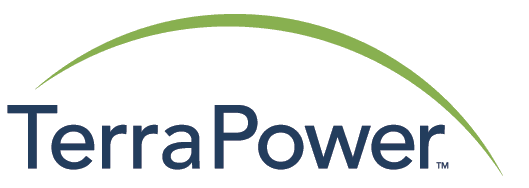
Natrium™ Nuclear Demonstration Plant Fuel Handling Simulation
Presented by Bryan Sardo, Senior Engineer
The NATRIUM Demonstration Plant will be built in Wyoming in the late 2020s. In order for a reactor to be operational, refueling outages are implemented to exchange fuel assemblies. The objective of the Natrium SIMIO refueling process is to simulate fuel assemblies during a refueling. The simulation is used to understand refueling outage requirements and impacting variables.

Using Simio's Dispatch Arrival Logic to Maximize Throughput While Meeting Service Levels
Presented by Jeff Brelsford, Co-Founder
Many manufacturers have two primary and competing objectives for their business. The first is to maximize system capacity by producing as much as possible within shorter time periods. The second is improving service levels, i.e., reducing lead times. Both of these objectives require production management techniques that are at odds with each other. Maximizing system capacity means reducing changeover and setup times which disregards the importance of due dates, which are important to maintaining service level agreements. This session discusses techniques for implementing these production management techniques using Simio’s built in dispatch logic and combining that with order selection via the Source object’s arrival logic.
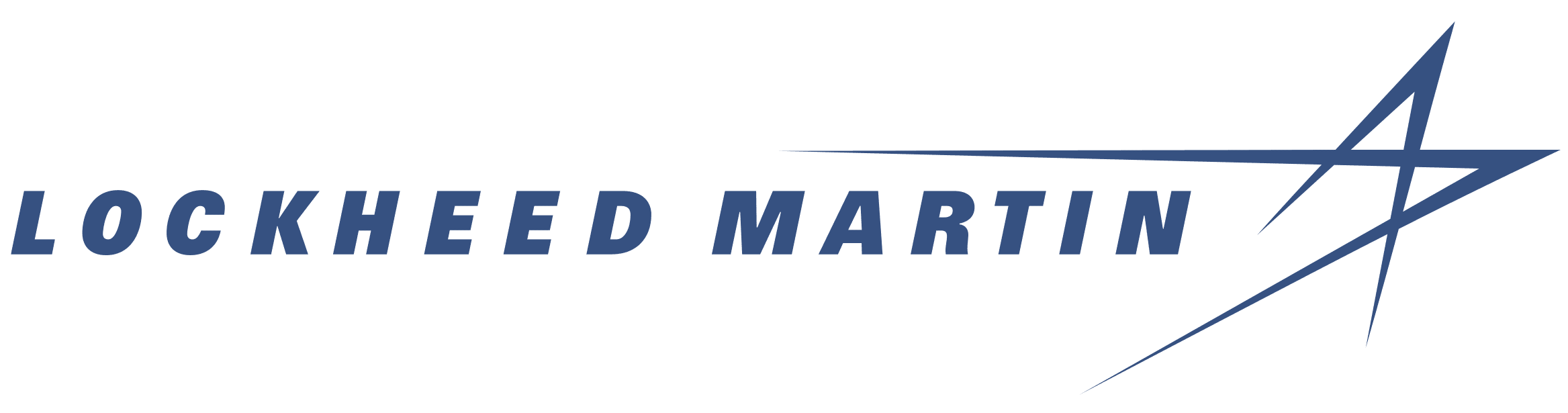
Large Component Modeling
Presented by Scott Swann, Modeling and Simulation Lead
Much of Lockheed Martin Aeronautics’ work involves assembling large components that take hundreds or thousands of man-hours to assemble in a single build area. During the time that a component is being assembled, many changes to the build area can occur, such as daily shift changes, monthly changes in manpower, allocation of personnel to work, part shortages, quality assurance checks and resulting rework or travelled work just to name a few. To accurately predict Aeronautics’ ability to meet contracted delivery commitments, these complexities need to be accounted for in the Simio models that are used to assess delivery confidence. This presentation will provide an overview of many of the modeling approaches and techniques Lockheed Martin Aeronautics used to more accurately model these complex behaviors.
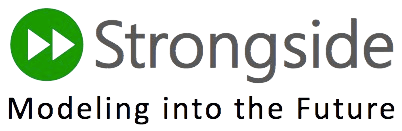
Using data-generated modeling and the Simio API to create a repeatable solution to a common modeling question
Presented by Bailey Kluczny, President
The overarching objective of this work was to expand access to simulation analysis and to improve decision support for internal customers. To support this goal, we explored using a system comprised of a web interface, database, and the Simio API in combination with data-generated modeling to simulate the use of a new type of equipment within a production facility. The system remotely runs a generalized Simio model driven by a set of data tables which define the facility’s key resources, locations, and workload. Any team member with access to the internal network can use the interface prompts to define their system and run a pre-built experiment to help assess whether an investment into the new equipment would likely have an adequate payback at their facility or not. This presentation describes some of the methods and lessons learned though the course of the work.
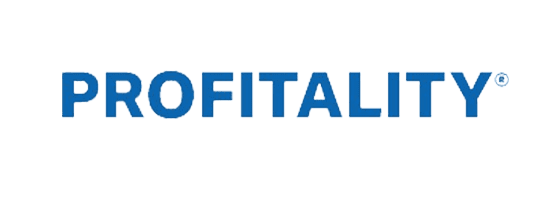
Drive-Thru Order Management: Tracking Metrics for Drive-Thru Optimization
Presented by Carlos Espinosa, Director & Shareholder Victor Cereijo, Senior Engineering Consultant
As the foodservice industry continues to evolve, restaurants are attempting to keep up with the rate of changes stemming from the influx of off-premise and drive-thru business. In drive-thru systems, managing “disruptors” to minimize delays at the window and order board has become more common as customer orders are more complex and their tolerance for waiting is reduced. Optimizing throughput and speed of service has come to the forefront of many concepts, which includes handling “exceptions” or “disruptors” with “Parking Procedures”. Computer simulation serves as a valuable, measurable method to identify areas of opportunity, and quickly turnaround the impact of solutions. Utilizing Simio, Profitality Labor Guru have created models of food service concepts to test and define optimal designs including equipment layout, labor deployments, and assembly procedures, but also includes unique decision logic that is used model parking procedures. Using decision trees and searches in the processes, the model determines changing outcomes to the scenarios tested. We will be discussing how the model’s core logic was created, such as order routing and process logic used to re-route orders based on variable factors as the model runs, and gathers unique data points that all restaurants should track in order to optimize their Drive-thru operations.
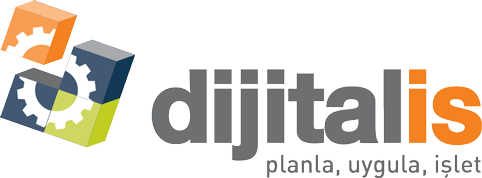
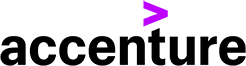
Improving food production operations using SIMIO
Presented by Guillermo Bonmati, Data Scientist Maria Servitja Robert, Data Scientist Manager
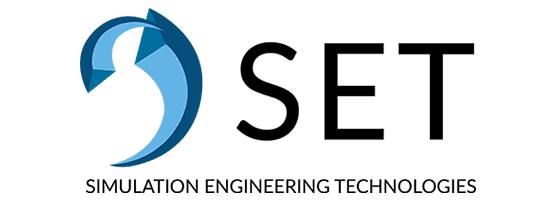
Representing the same system using discrete entities, flow, or tokens
Presented by Zamri Combrinck, Industrial Engineer Hulda Viljoen, Industrial Engineer
Determining the best modelling approach to simulate a real-world process can often be tricky – the most obvious approach is not necessarily the only option, nor the most effective. A common mistake is getting caught up in the material being modelled (for instance, discrete entities) and, therefore, thinking that a discrete simulation model is the only way to go.
To illustrate this concept, a simple bottling plant simulation is constructed using three different approaches, each varying in the level of detail. The first approach uses discrete modelling to represent each bottle as a unique entity, while the second method approximates the movement of volumes of bottles as a continuous flow. It is possible to take it one step further and remove all entities from the model by using Simio’s token functionality and processes to control what would happen to the bottles without having any entities in the model.
These three simulation approaches are compared in outcomes achieved in terms of accuracy and then also on run speed and development time required. Each method offers a different level of insight, and successfully identifying which is sufficient for your purposes could save significant development hours.
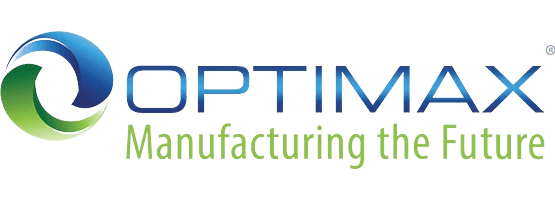
Reducing Agnostic Part Takt Time by Using Process Design in Simio
Presented by John Gilmore, Process Automation Engineer
Optimax builds the optics behind the latest breakthrough technologies in aerospace, defense, and consumer electronics. Optimax has developed highly engineered processes as well as superior fabrication and coating capabilities for advanced optics for space applications, high power directed energy systems, and semiconductor industries. Simio is being used to design a part agnostic process that reduces takt time by roughly 600%. The discrete stochastic work flow and table design structure is why Optimax decided to use Simio over most of its competitors. The simulation was developed for part agnosticism where the process equations have been designed to allow for multiple part specifications and machine configurations. Utilizing both the table structure embedded in Simio; along with its experimental features, Optimax is able to test multiple different process structures and part flows resulting in a robust dynamic facility design.
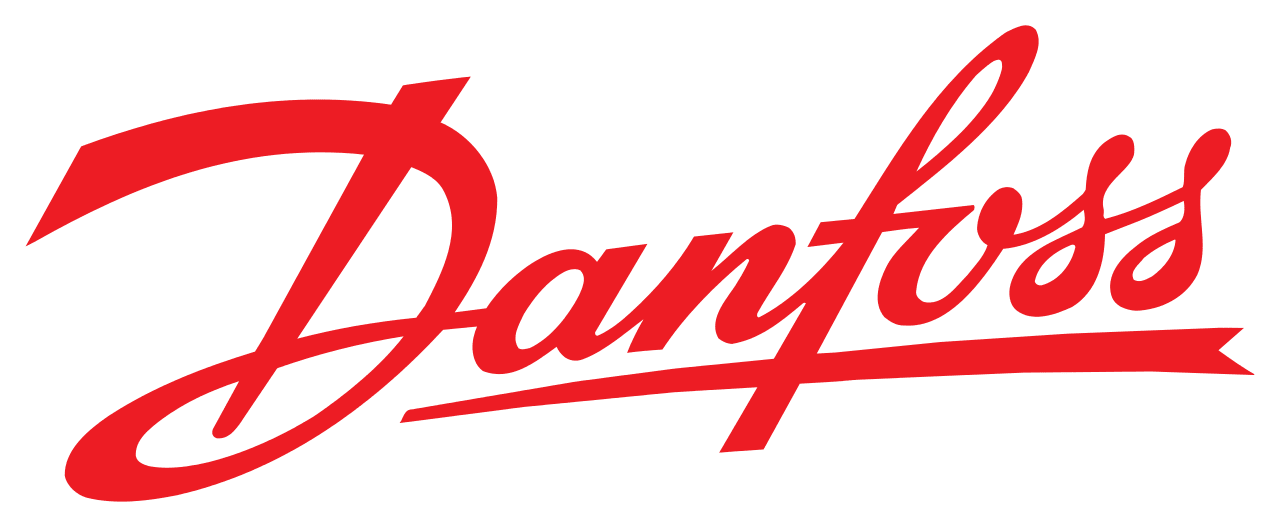
Discrete event simulation for paint, packaging, and receiving processes using Simio
Presented by Mohamed Eldakroury, Manufacturing Engineer Amy Walczyk, Process Engineering Intern
Danfoss engineers the technologies that enable the world of tomorrow to do more with less. We meet the growing need for infrastructure, food supply, energy efficiency, and climate-friendly solutions. At Danfoss power solutions, we use Simio across many areas of the organization. We use simulation in evaluating the performance of current assembly lines, paint lines, and our receiving department. In today’s environment, where uncertainty and volatility impact businesses, quick responses to global economic changes are often needed. Simio has helped us to react quickly to those changes. In recent years, we used Simio to make decisions regarding expansion projects that would significantly impact quality, delivery, and safety. We will share some of those examples, and how some Simio features like data tables and add-on processes helped us develop simulation models that are very close to reality. We will also share some challenges and lessons learned from developing small and large-scale simulation models.
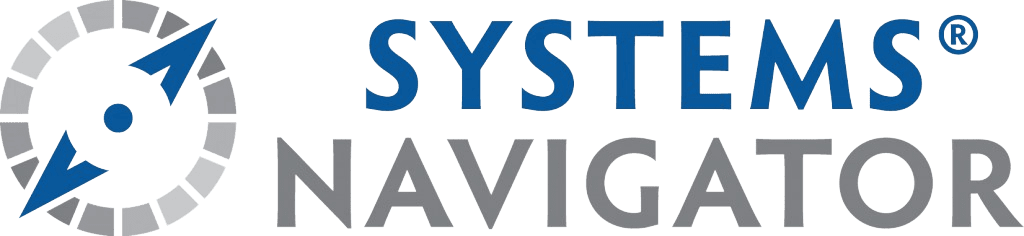
Carbon capture and storage (CCS)
Presented by Rienk Bijlsma, Founder & CEO
Carbon capture and storage (CCS) is the process of capturing waste carbon dioxide (CO2) usually from large point sources, transporting it to a storage site, and depositing it where it will not enter the atmosphere, normally an underground geological formation. Due to the high investment and operating costs for CCS supply chains, this technology has not been widely adopted, but this is bound to change as emission rights become more expensive. Pipelines in combination with marine transport of liquified CO2 are seen as an effective solution for CCS that is both flexible, as well as capable to economically move large volumes to a storage site. Accurately predicting the networks costs is crucial in making the best design decisions for the supply chain. Given the network interaction, variability, storage requirements and operating policies, simulation is a great technology to accurately predict the supply chain cost range for various design choices.
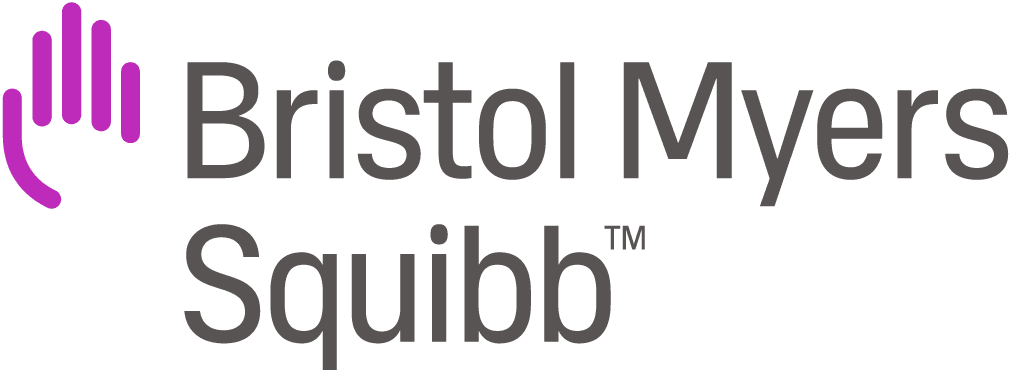
Cell Therapy Facility Modeling
Presented by Alexander Wessner, Process Engineer; Process Equipment and Engineering
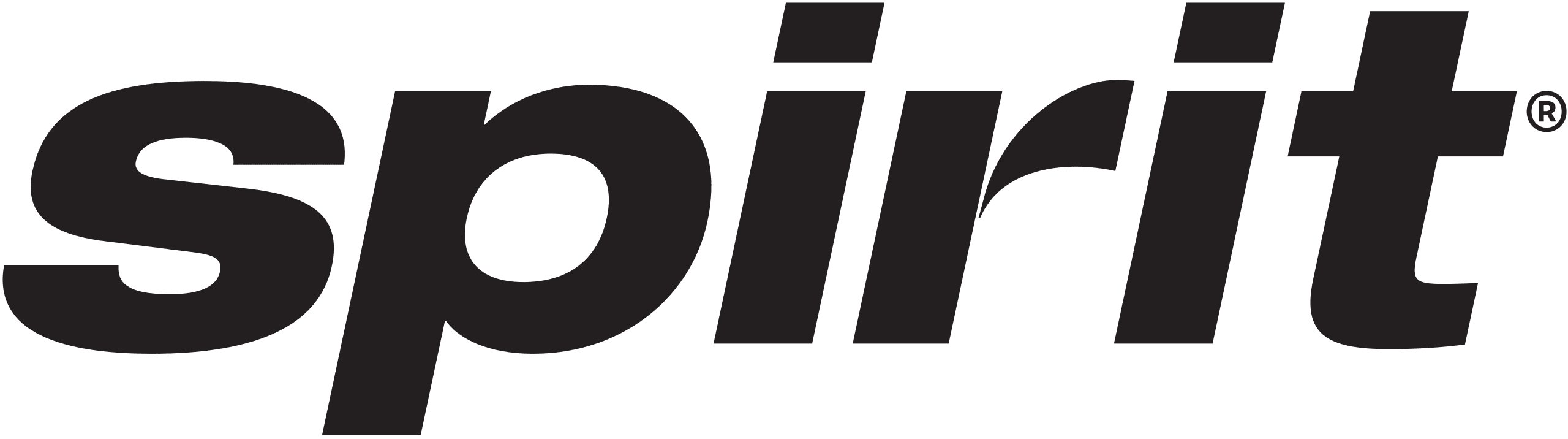
Addressing Lobby Check-In Challenges with Simio
Presented by Maria Carolina Diaz, Business Process Optimization Manager
Covid-19 caught the whole world by surprise. No industry can say it didn’t get impacted by COVID; everyone got impacted in varying degrees. In order to survive the crisis, companies had to be quick in order to respond to WHO recommendations, and consumer expectations. Airlines were no different! First, airlines had to downsize their operation in order to minimize the cash burn through those months were travel demand virtually disappeared and, then, when it restarted, airlines had the challenge to readjust the size of their operation to be prepared to respond to those guests coming back and regaining their confidence for traveling. An area that was very much affected was the area of check in given that every country has different laws and requirements when it comes to accepting the flying public into their borders; additionally, given that these requirements keep changing every day, and no one had any baseline for dealing with such situation, scheduling personnel for this situation became a problem, affecting the time it takes guests to be checked in for their flights. In this presentation, I will walk you through what were the problems our guests were facing, the challenges that we faced as an airline when trying to solve this problem for our guests, and lastly how we used a simulation model to help us determine the ideal number of people serving our guests at our lobby in our busiest airport.
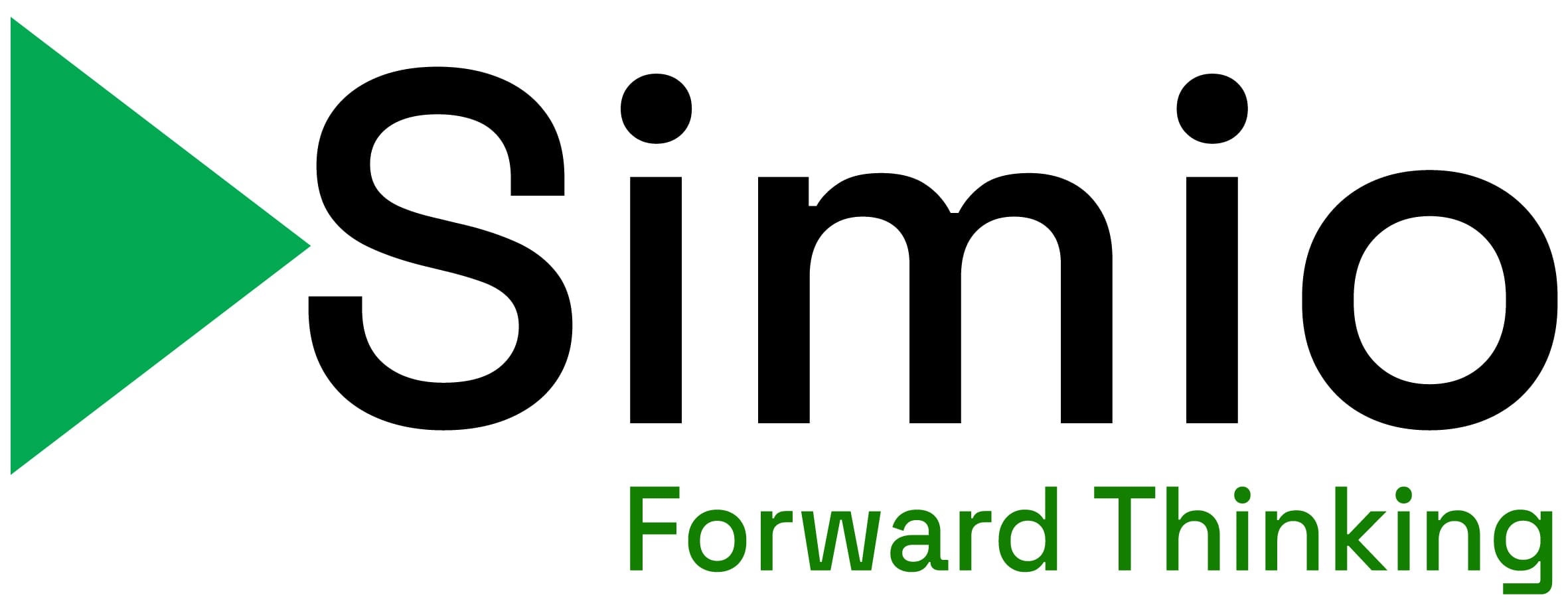
Tips and Tricks to Enhance Your Model to be Data Generated and Data Driven
Presented by Elizabeth Millar, Applications Engineer
Large volumes of data are often available to build and drive a simulation model. Efficient and appropriate use of this data is critical to model success. When a project begins, the path forward using a data generated or data driven approach may not be clear. However, it is never too late to change to a data-oriented strategy. Simio is equipped with features that will minimize re-work and ease this transition. This presentation will review some tips and tricks on how to shift the modeling approach in an existing model from traditional methods to data driven and data generated. A manufacturing example model will be enhanced through building and referencing Data Tables and Table Relationships, leveraging custom objects, and utilizing shortcut actions. The result is a model that is logically organized with data and has easy options to expand through data.
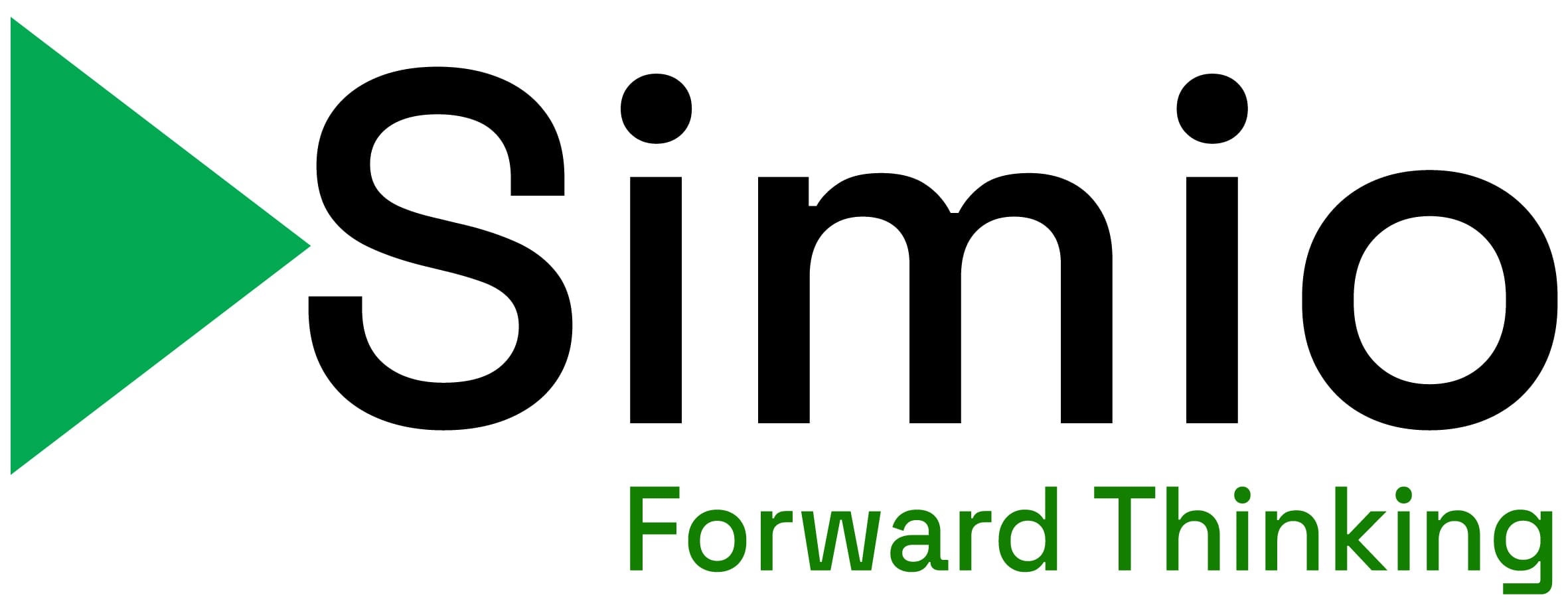
Neural Networks in Simio: A Supply Chain Example
Presented by Rylan Carney, Applications Engineer
Simio’s neural network features present many new opportunities in the realm of AI, including the use of discrete-event simulation (DES) to generate synthetic training data for neural networks or other machine learning algorithms. Many machine learning algorithms require large quantities of training data, which DES can provide. Additionally, the input parameters of DES models can be adjusted to provide balanced training data to create robust machine learning models. Beyond synthetic training data, DES models can also be used to evaluate machine learning models before production deployment. In this presentation, Simio will be used to generate training data, train a neural network, use a neural network to route orders, and evaluate the impact of neural network routing in a supply chain.
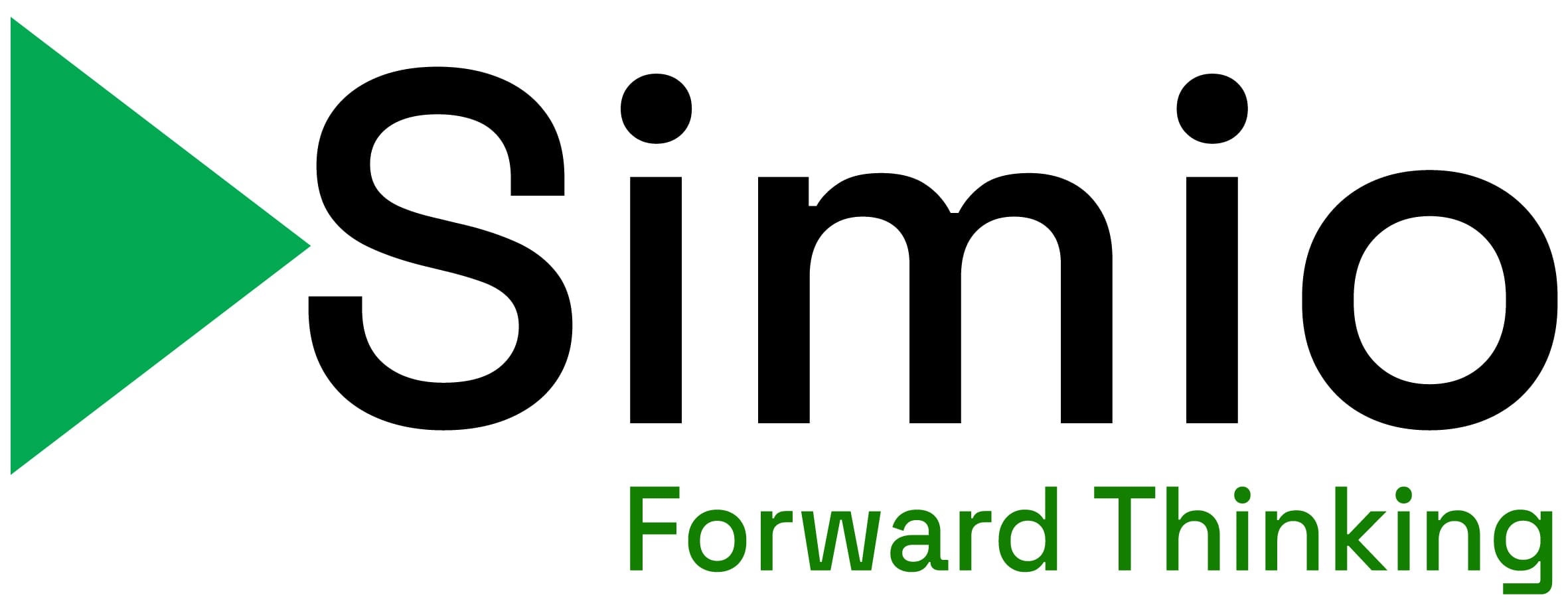
Make, Transport, and Replenish Template
Presented by Glen Wirth, Solutions Architect
This template model highlights the use of transporters for material movements and material replenishment policies uses in scheduling applications and shows some of the best practices that Simio has identified. Specifically, we will highlight how the schema and processes were built to be completely data driven while representing some of the unique aspects modeling material flow. The template not only is able to schedule resources, workers, and materials, it also models the transporters needed to move raw, intermediate, and finished materials.
The table schema in the model is based on the ISA-95 and ISA-88 standards. The model is setup to model at a very detailed level. In addition, the transporters can be modeled as “cart” where workers are needed to move the vehicles, or the transporters can be modeled as “AMRs” with battery charging and movement in free space movement along a path with collision avoidance. The template also is setup to work as a real-time dispatcher of work. The model can be setup to send commands to vehicle to begin transport assignments and to update the location of the vehicle in the model during its run using IIOT connectivity.
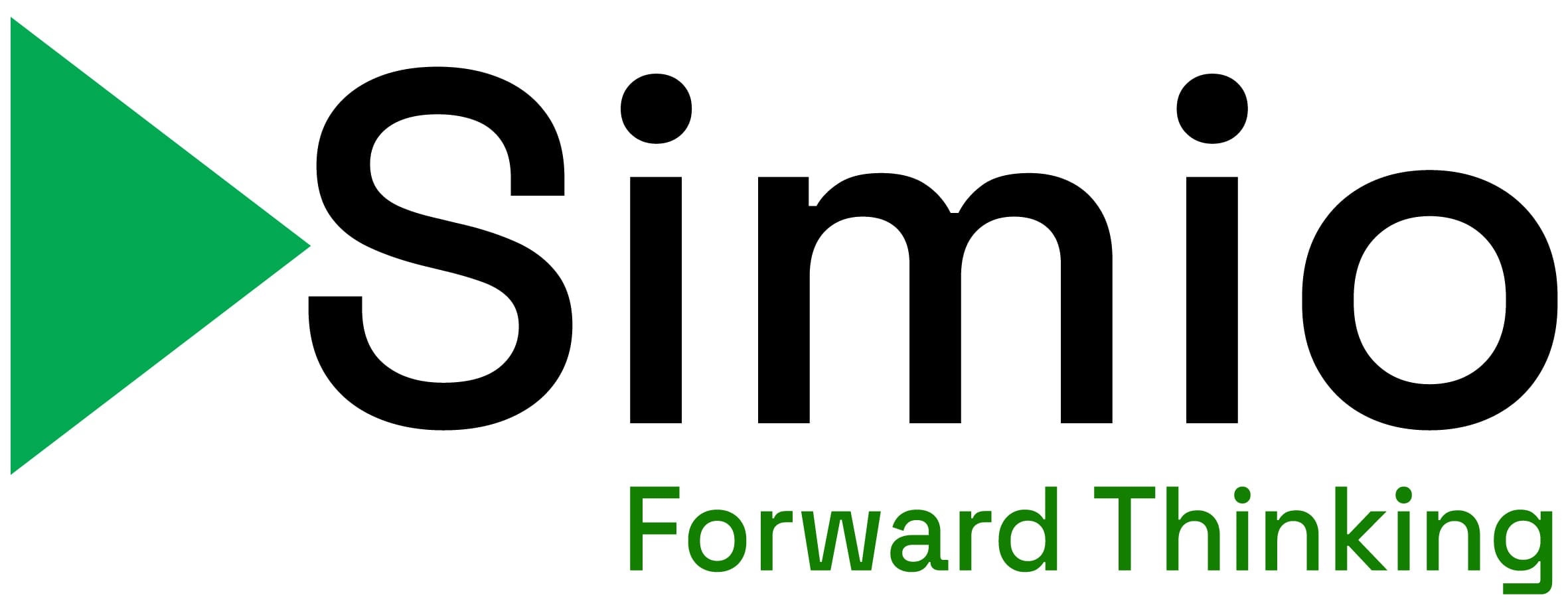
Mix, Tank, Fill, and Template
Presented by Devdatta Deo, Senior Engineer
There are many systems that rely on a limited buffer space (i.e., tanks) in between upstream and downstream resources. Properly representing the capacity of the tanks requires an event calendar to track flows into and out of these tanks. Simio will show the best practices we’ve identified to capture these constraints in a data driven framework. Some unique constraints include tank cleaning, limited connectivity (i.e., many to many, but not all to all), and efficient campaigning with process logic (e.g., how to make Simio objects wait to avoid changeovers) as well as some logic optimization techniques to keep compute times low.
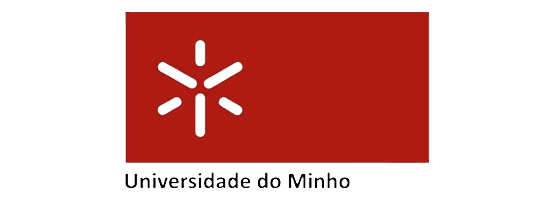
Manufacturing Flexibility in Market Fluctuation
Presented by Marcelo Henriques, Researcher and Invited Lecturer
In order to answer to market fluctuation, organizations have resorting to manufacturing system design, as a way to improve their manufacturing systems’ flexibility. However, this is a very hard and complex process. Fortunately, Discrete Event Simulation (DES) can help in this regard. However, DES is not yet a ubiquitous tool in this field.
To help in this regard, and in order to (1) reduce the time it takes DES practitioners to model a manufacturing system, and (2) make simulation models which are both standardize on their modelling approach and generic on their behaviour, an automatic generator of simulation models can be used, which is only viable through the use of simulation models.
This presentation focuses on the development of simulation objects, guarantying they are generic, using sub-classing to improve efficiency on future modelling, and the visualization aspect of simulation.
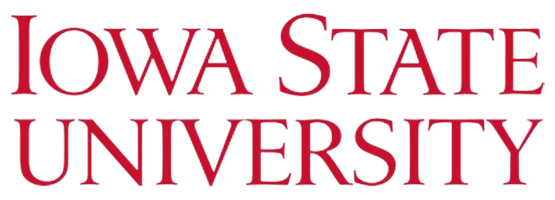
Analytical Decision Making with a Digital Twin
Presented by Cameron MacKenzie, Assistant Professor Zhouyi Zhao, Ph.D. Student Sylvia Knight, Masters Student
A digital twin should enable a company to make real-time decisions by enabling them to understand, simulate, and visualize how those decisions will impact their manufacturing and production schedules. This talk will discuss how we are exploring the potential use of Simio as a digital twin with a manufacturing company to help the company make decisions with their production systems. First, we identify some scenarios or uses cases that frequently occur in production systems and determine to what extent a digital twin can help address those scenarios. Second, we create those scenarios in Simio using some simplified simulations of production systems and analyze how a digital twin within Simio can benefit decision making. This talk will focus on scenarios for condition-based maintenance and simulating production starting from the current state of production in the facility.
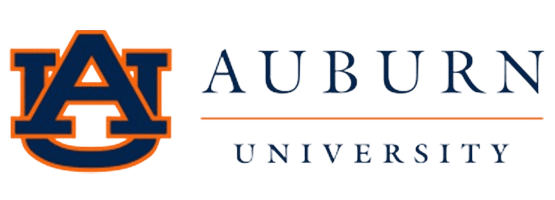
A Framework for Integrating Gurobi Optimization within Simio
Presented by Grant Romine, Ph.D. Student
Within discrete-event simulation, decisions are made using rule sets (such as dispatching) that are defined before each run. Some real-world systems have decisions that are difficult to model using this approach, which can lead to decisions that are locally “best,” but are myopic with regards to the entire system. This presentation presents a technology framework that integrates the Gurobi optimization solver within a Simio model. Periodically during a run, Simio executes Gurobi to make a decision that considers the current state of the model. The result is then used inside the Simio model as it continues its run. Integrating these two technologies provides a modeling approach that retains both optimization’s global decision-making and discrete-event simulation’s ability to handle uncertainty and complexity.